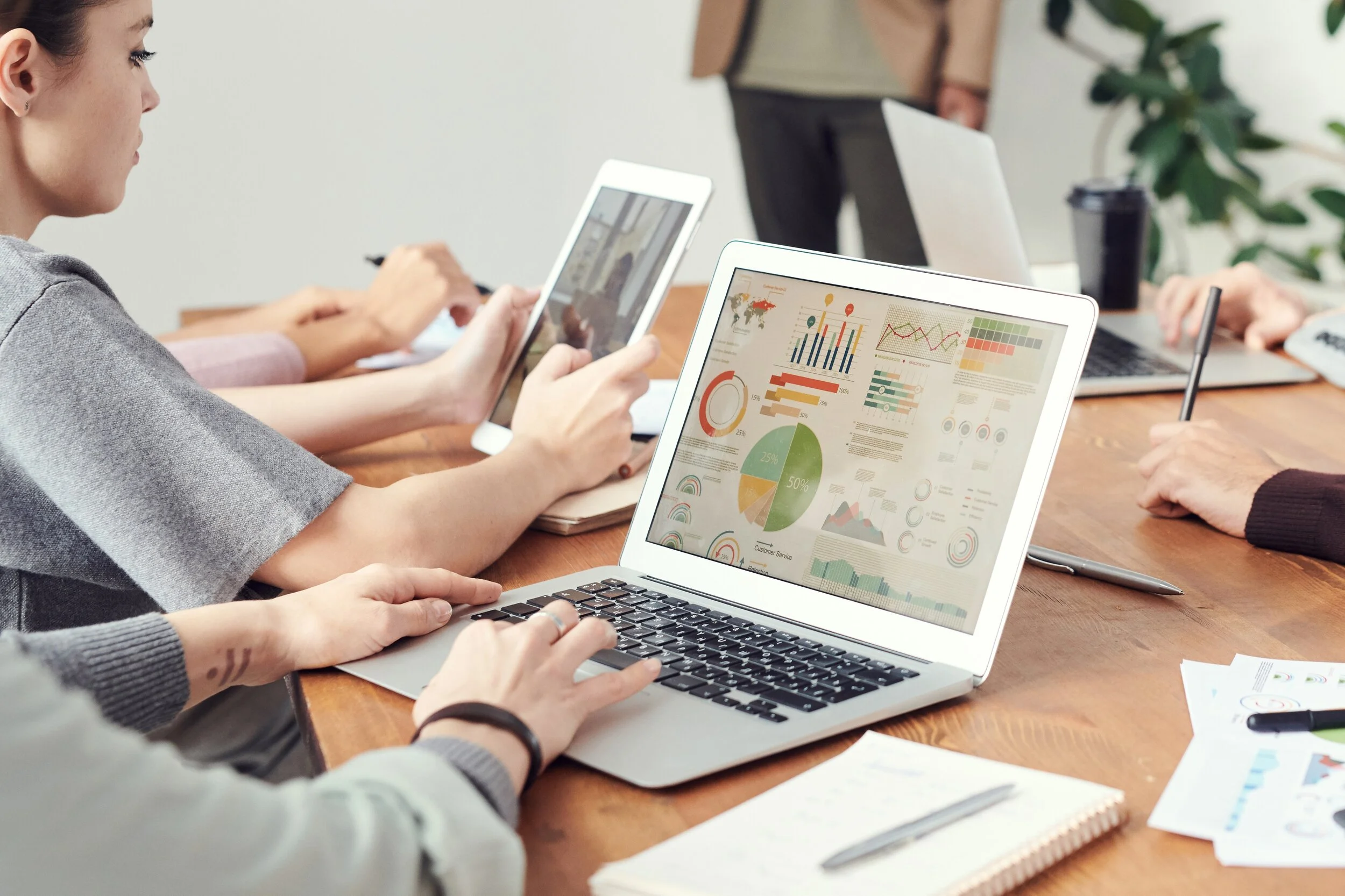
CASE STUDY
Hybrid Data Modeling for When Traditional Dimensional & Normalized Data Models Become Operationally Unsustainable
SERVICES
THE CHALLENGE
Traditional & normalized data models become operationally unsustainable
A regional payer organization initiated an enterprise-wide data consolidation and integration effort to support their objective of deploying a unified, conformed, and trusted source of data for reporting and analysis.
The organization had data with significant overlap across multiple functional silos. Reconciling across multiple sources of data had become an inefficient and unnecessary effort fraught with risk.
The challenge with consolidating and integrating data is architecting a target data model that adapts quickly, models the business accurately, and scales in accordance with business needs.
Unifying and conforming data from across the enterprise requires a carefully considered construct in which to organize the resulting data asset. Traditionally, practitioners have employed dimensional data modeling and even some form of normalized modeling to design the target destination for integrated data. However, both approaches have their limitations.
Reconciling across multiple sources of data had become an inefficient and unnecessary effort fraught with risk.
OUR SOLUTION
Hybrid data model approach with hub, satellite, and link constructs
We started by examining the nature of the data that the organization has been acquiring, organizing, analyzing, and governing over time. We juxtaposed that with information needs and the organization’s approach to producing such information over the same time period.
We found that the complexity and velocity of information needs have increased significantly in recent times, and along with this increase we found the emergence of independent information silos and corresponding increases in IT support spend to keep up with demand.
TOOLKIT
Root Cause Analysis
User Persona Journey
Data Model Design Mapping
The traditional data warehouse architecture and by extension its downstream information marts needed costly reengineering and reloads to satisfy demand, and the root cause of this was the lack of robustness of the existing dimensional and normalized data model in the face of incoming changes and volume of data.
To capture data growth in a standardized and usable model across department, we mapped and delivered a hybrid data model approach with hub, satellite, and link constructs.
THE RESULTS
By mapping a hybrid data model approach with hub, satellite, and link constructs, we delivered design aspects addressing all limitations of the previous data model, including:
Cascading impact of changes in data content, relationships, and business rules
Load dependencies and late arriving data
Immutability principle of data warehousing and the preservation of historical content and representation
Compliance with regulatory & internal guidelines and auditability to enable attestation